Rudolf Grünbichler – Graz University of Technology, Faculty of Mechanical Engineering and Economic Sciences, Institute of Business Economics and Industrial Sociology, Kopernikusgasse 24/II, 8010 Graz, Austria
Raphael Krebs – Graz University of Technology, Faculty of Mechanical Engineering and Economic Sciences, Institute of Business Economics and Industrial Sociology, Kopernikusgasse 24/II, 8010 Graz, Austria
7th International Scientific-Business Conference – LIMEN 2021 – Leadership, Innovation, Management and Economics: Integrated Politics of Research – SELECTED PAPERS, Online/virtual, December 16, 2021, published by the Association of Economists and Managers of the Balkans, Belgrade; Printed by: SKRIPTA International, Belgrade, ISBN 978-86-80194-53-0, ISSN 2683-6149, DOI: https://doi.org/10.31410/LIMEN.S.P.2021
Keywords:
Corporate insolvencies;
Corporate bankruptcy;
Artificial intelligence;
Small and Medium-sized
Companies
Abstract
Digitization in enterprises enables the application of artificial intelligence, especially machine learning. One area of use for artificial intelligence is in the creation of an insolvency forecast for companies. With a literature review, the current status on the usage of artificial intelligence in insolvency forecasting is presented. For this purpose, the two databases Scopus and Web of Science are searched for scientific papers on the topic of artificial intelligence and corporate insolvencies to get an up-to-date impression of the status quo. A particular focus is placed on small and medium-sized companies. It is shown that artificial intelligence methods provide better results compared to classical methods. The research reveals that the most important algorithms related to the prediction of potential corporate insolvency are artificial neural networks, decision trees and support vector machines as well as hybrid models.
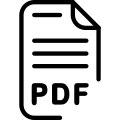
Download file
LIMEN Conference
Creative Commons Non Commercial CC BY-NC: This article is distributed under the terms of the Creative Commons Attribution-Non-Commercial 4.0 License (https://creativecommons.org/licenses/by-nc/4.0/) which permits non-commercial use, reproduction and distribution of the work without further permission.
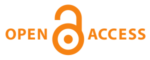
References
Abdelmoula, A. K. (2015). Bank Credit Risk Analysis with K-Nearest-Neighbor Classifier: Case of Tunisian Banks. Journal of Accounting and Management Information Systems, 14(1), 79–106. https://ideas.repec.org/a/ami/journl/v14y2015i1p79-106.html
Addo, P. M., Guegan, D., & Hassani, B. (2018). Credit Risk Analysis Using Machine and Deep Learning Models. Risks 2018, Vol. 6, Page 38, 6(2), 38. https://doi.org/10.3390/ RISKS6020038
Alaka, H., Oyedele, L., Owolabi, H., Akinade, O., Bilal, M., & Ajayi, S. (2019). A Big Data Analytics Approach for Construction Firms Failure Prediction Models. IEEE Transactions on Engineering Management, 66(4), 689–698. https://doi.org/10.1109/TEM.2018.2856376
Angelini, E., di Tollo, G., & Roli, A. (2008). A neural network approach for credit risk evaluation. Quarterly Review of Economics and Finance, 48(4), 733–755. https://doi.org/10.1016/j. qref.2007.04.001
Arora, N., & Kaur, P. D. (2020). A Bolasso based consistent feature selection enabled random forest classification algorithm: An application to credit risk assessment. Applied Soft Computing, 86, 105936. https://doi.org/10.1016/J.ASOC.2019.105936
Bahrammirzaee, A. (2020). A comparative survey of artificial intelligence applications in finance: artificial neural networks, expert system and hybrid intelligent systems, Neural Computing & Applications, 19, pp. 1165-1195.
Bao, Y., Hilary, G. & Ke, B. (2022). Artificial Intelligence and Fraud Detection, in: Babich, V., Birge, J. R. & Hilary, G. (eds) Innovative Technology at the Interface of Finance and Operations. Springer Series in Supply Chain Management, vol. 11, Springer Gabler Verlag.
Bekhet, H. A., & Eletter, S. F. K. (2014). Credit risk assessment model for Jordanian commercial banks: Neural scoring approach. Review of Development Finance, 4(1), 20–28. https://doi. org/10.1016/J.RDF.2014.03.002
Boughaci, D., Alkhawaldeh, A. A. K., Jaber, J. J., & Hamadneh, N. (2021). Classification with segmentation for credit scoring and bankruptcy prediction. Empirical Economics, 61(3), 1281–1309. https://doi.org/10.1007/S00181-020-01901-8
Burda, A., Cudek, P., & Hippe, Z. S. (2013). Profiieseeker – Early warning system for predicting economic situation of small and medium enterprises. 2013 6th International Conference on Human System Interactions, HSI 2013, 398–400. https://doi.org/10.1109/HSI.2013.6577854
Chamoni, P. & Gluchowski, P. (2017). Business Analytics – State of the Art, Controlling & Management Review, 4, 2017, pp. 8-17.
Ciampi, F., & Gordini, N. (2013). Small Enterprise Default Prediction Modeling through Artificial Neural Networks: An Empirical Analysis of Italian Small Enterprises. Journal of Small Business Management, 51(1), 23–45. https://doi.org/10.1111/j.1540-627X.2012.00376.x
Ciampi, F., Giannozzi, A., Marzi, G., & Altman, E. I. (2021). Rethinking SME default prediction: a systematic literature review and future perspectives. Scientometrics, 126(3), 2141– 2188. https://doi.org/10.1007/s11192-020-03856-0
Di Vaio, A., Palladino, R., Hassan, R. & Escobar, O. (2020). Artificial Intelligence and business models in the sustainable development goals perspective: A systematic literature review, Journal of Business Research, 121, pp. 283-314.
Doubek, C., Exler, M. & Situm, M. (2016). Aktueller Stand von Früherkennungssystemen. Krisen-, Sanierungs- und Insolvenzberatung 5/2016, pp. 204–210.
Fantazzini, D., & Figini, S. (2008). Random Survival Forests Models for SME Credit Risk Measurement. Methodology and Computing in Applied Probability 2008 11:1, 11(1), 29– 45. https://doi.org/10.1007/S11009-008-9078-2
Gentsch, P. (2019). Business KI verändert Unternehmen und Märkte, Controlling & Management Review, 4, 2019, pp. 24-32.
Giannopoulos, V., & Aggelopoulos, E. (2019). Predicting SME loan delinquencies during recession using accounting data and SME characteristics: The case of Greece. Intelligent Systems in Accounting, Finance and Management, 26(2), 71–82. https://doi.org/10.1002/ ISAF.1456
Gregova, E., Valaskova, K., Adamko, P., Tumpach, M., & Jaros, J. (2020). Predicting Financial Distress of Slovak Enterprises: Comparison of Selected Traditional and Learning Algorithms Methods. Sustainability 2020, Vol. 12, Page 3954, 12(10), 3954. https://doi. org/10.3390/SU12103954
Han, J. J. & Kim, H.-J. (2021). Stock price prediction using multiple valuation methods based on artificial neural networks for KOSDAQ IPO companies, Investment Analysts Journal, 50 (1), pp. 17-31.
Huang, X., Liu, X., & Ren, Y. (2018). Enterprise credit risk evaluation based on neural network algorithm. Cognitive Systems Research, 52, 317–324. https://doi.org/10.1016/J. COGSYS.2018.07.023
J, U., Metawa, N., Shankar, K., & Lakshmanaprabu, S. K. (2020). Financial crisis prediction model using ant colony optimization. International Journal of Information Management, 50, 538–556. https://doi.org/10.1016/J.IJINFOMGT.2018.12.001
Koropp, C. & Treitz, R. (2019). Performance Management mit Advanced Analytics, Controlling & Management Review, 6, pp. 32-38.
KSV1870, Insolvenzstatistik 2021, https://www.ksv.at/insolvenzstatistik/insolvenzstatistik-2021-final, accessed on: 15.01.2022.
Li, K., Niskanen, J., Kolehmainen, M., & Niskanen, M. (2016). Financial innovation: Credit default hybrid model for SME lending. Expert Systems with Applications, 61, 343–355. https://doi.org/10.1016/J.ESWA.2016.05.029
Lui, A. K. H., Lee, M. C. M. & Ngai, E. W. T. (2022). Impact of Artificial Intelligence investment on Firm Value, Annals of Operations Research, 308 (1-2), pp. 373-388. 10.1007/ s10479-020-03862-8
Malakauskas, A., & Lakstutiene, A. (2021). Financial distress prediction for small and medium enterprises using machine learning techniques. Engineering Economics, 32(1), 4–14. https://doi.org/10.5755/j01.ee.32.1.27382
Mertens, P. & Barbian, D. (2019). Erreicht Künstliche Intelligenz auch das Controlling?, Controlling & Management Review, 4, pp. 8-17.
Mishra, A. N. & Pani, A. K. (2020). Business value appropriation roadmap for artificial intelligence, Vine Journal of Information and Knowledge Management Systems, 51 (3), pp. 353-368.
Mittal, S., Gupta, P., & Jain, K. (2011). Neural network credit scoring model for micro enterprise financing in India. Qualitative Research in Financial Markets, 3(3), 224–242. https://doi. org/10.1108/17554171111176921/FULL/XML
Moscatelli, M., Parlapiano, F., Narizzano, S., & Viggiano, G. (2020). Corporate default forecasting with machine learning. Expert Systems with Applications, 161, 113567. https://doi. org/10.1016/J.ESWA.2020.113567
Nanni, L., & Lumini, A. (2009). An experimental comparison of ensemble of classifiers for bankruptcy prediction and credit scoring. Expert Systems with Applications, 36(2), 3028– 3033. https://doi.org/10.1016/J.ESWA.2008.01.018
Oliveira, M. D. N. T., Ferreira, F. A. F., Pérez-Bustamante Ilander, G. O., & Jalali, M. S. (2017). Integrating cognitive mapping and MCDA for bankruptcy prediction in small- and medi um-sized enterprises. Journal of the Operational Research Society 2017 68:9, 68(9), 985– 997. https://doi.org/10.1057/S41274-016-0166-3
Plastino, E. & Purdy, M. (2018). Game Changing value from Artificial Intelligence: eight strategies, Strategy & Leadership, 46 (1), pp. 16-22.
Qu, Y., Quan, P., Lei, M., & Shi, Y. (2019). Review of bankruptcy prediction using machine learning and deep learning techniques. Procedia Computer Science, 162, 895–899. https:// doi.org/10.1016/j.procs.2019.12.065
Reis, C., Ruivo, P., Oliveira, T. & Faroleiro, P. (2020). Assessing the drivers of machine learning business value, Journal of Business Research, 117, pp. 232-243.
Sigrist, F., & Hirnschall, C. (2019). Grabit: Gradient tree-boosted Tobit models for default prediction. Journal of Banking & Finance, 102, 177–192. https://doi.org/10.1016/J.JBANKFIN.2019.03.004
Snyder, H. (2019). Literature Review as a research methodology: An overview and guidelines, Journal of Business Research, 104, pp. 333-339.
Teles, G., Rodrigues, J. J. P. C., Rabêlo, R. A. L., & Kozlov, S. A. (2021). Comparative study of support vector machines and random forests machine learning algorithms on credit operation. Software: Practice and Experience, 51(12), 2492–2500. https://doi.org/10.1002/SPE.2842
Tsai, C. F., & Wu, J. W. (2008). Using neural network ensembles for bankruptcy prediction and credit scoring. Expert Systems with Applications, 34(4), 2639–2649. https://doi. org/10.1016/J.ESWA.2007.05.019
Tseng, F. M., & Hu, Y. C. (2010). Comparing four bankruptcy prediction models: Logit, quadratic interval logit, neural and fuzzy neural networks. Expert Systems with Applications, 37(3), 1846–1853. https://doi.org/10.1016/j.eswa.2009.07.081
Wamba-Taguimdje, S.-L., Wamba, S. F., Kamdjoug, J. R. K. & Wanko, C. E. T. (2020). Influence of artificial intelligence (AI) on firm performance: the business value of AI-based transformation projects, Business Process Management Journal, 26 (7), pp. 1893-1924.
Wang, G., & Ma, J. (2011). Study of corporate credit risk prediction based on integrating boosting and random subspace. Expert Systems with Applications, 38(11), 13871–13878. https:// doi.org/10.1016/J.ESWA.2011.04.191
Wang, G., & Ma, J. (2012). A hybrid ensemble approach for enterprise credit risk assessment based on Support Vector Machine. Expert Systems with Applications, 39(5), 5325–5331. https://doi.org/10.1016/J.ESWA.2011.11.003
Wang, G., Hao, J., Ma, J., & Jiang, H. (2011). A comparative assessment of ensemble learning for credit scoring. Expert Systems with Applications, 38(1), 223–230. https://doi.org/10.1016/J. ESWA.2010.06.048
Wang, G., Ma, J., & Yang, S. (2014). An improved boosting based on feature selection for corporate bankruptcy prediction. Expert Systems With Applications, 41, 2353–2361. https:// doi.org/10.1016/j.eswa.2013.09.033
Wang, G., Ma, J., Huang, L., & Xu, K. (2012). Two credit scoring models based on dual strategy ensemble trees. Knowledge-Based Systems, 26, 61–68. https://doi.org/10.1016/J.KNOSYS.2011.06.020
Webster, J. & Watson R. T. (2002). Analyzing the Past to Prepare for the Future: Writing a Literature Review, MIS Quarterly, 26 (2), pp. xiii-xxiii.
Wright, R. W., Brand, R. A., Dunn, W. & Spindler, K. P. (2007). How to Write a Systematic Review, Clinical Orthopaedics and related Research, 455, pp. 23-29.
Zhu, Y., Xie, C., Sun, B., Wang, G. J., & Yan, X. G. (2016). Predicting China’s SME Credit Risk in Supply Chain Financing by Logistic Regression, Artificial Neural Network and Hybrid Models. Sustainability 2016, Vol. 8, Page 433, 8(5), 433. https://doi.org/10.3390/SU8050433
Zhu, Y., Xie, C., Wang, G. J., & Yan, X. G. (2016). Predicting China’s SME Credit Risk in Supply Chain Finance Based on Machine Learning Methods. Entropy 2016, Vol. 18, Page 195, 18(5), 195. https://doi.org/10.3390/E18050195
Zhu, Y., Xie, C., Wang, G. J., & Yan, X. G. (2017). Comparison of individual, ensemble and integrated ensemble machine learning methods to predict China’s SME credit risk in supply chain finance. Neural Computing and Applications, 28, 41–50. https://doi.org/10.1007/ s00521-016-2304-x
Zhu, Y., Zhou, L., Xie, C., Wang, G. J., & Nguyen, T. v. (2019). Forecasting SMEs’ credit risk in supply chain finance with an enhanced hybrid ensemble machine learning approach. International Journal of Production Economics, 211, 22–33. https://doi.org/10.1016/j. ijpe.2019.01.032