Dijana Vuković – University of North, Jurja Križanića 31b, 42000 Varaždin, Croatia
Sara Slamić Tarade – Zagreb University of Applied Sciences, Vrbik 8, 10000 Zagreb, Croatia
Keywords:
Brand significance;
Brand value;
Natural Language Processing;
Topic modeling;
Semantic Brand Score
Abstract: Determining the value of brands and comparing them by analyzing key elements such as identity, image and value is important for marketing measures and the branding of products or services. This paper presents research findings based on innovative methods for determining the significance and value of sports footwear brands. Natural Language Processing (NLP) techniques are used to analyze extensive text content collected from sports footwear-related websites. To determine the most relevant sports footwear brands, NLP techniques are used for topic modeling based on the Latent Dirichlet Allocation (LDA) method. LDA is an unsupervised method used to determine the topics addressed in the analyzed texts by extracting the most significant words in these topics. The importance of these identified brands in the text corpus is determined using the Semantic Brand Scores method, which uses graph theory to determine the importance of the brand in the text corpus based on three dimensions: prevalence, diversity and connectivity.
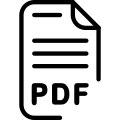
Download file
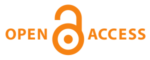
LIMEN Conference
9th International Scientific-Business Conference – LIMEN 2023 – Leadership, Innovation, Management and Economics: Integrated Politics of Research – CONFERENCE PROCEEDINGS, Hybrid (Graz University of Technology, Graz, Austria), December 7, 2023
LIMEN Conference Proceedings published by the Association of Economists and Managers of the Balkans, Belgrade, Serbia
LIMEN Conference 2023 Conference Proceedings: ISBN 978-86-80194-78-3, ISSN 2683-6149, DOI: https://doi.org/10.31410/LIMEN.2023
Creative Commons Non Commercial CC BY-NC: This article is distributed under the terms of the Creative Commons Attribution-Non-Commercial 4.0 License (https://creativecommons.org/licenses/by-nc/4.0/) which permits non-commercial use, reproduction and distribution of the work without further permission.
Suggested citation
Vuković, D., & Slamić Tarade, S. (2023). Investigating the Value of Sports Footwear Brands using Natural Language Processing Methods. In V. Bevanda (Ed.), International Scientific-Business Conference – LIMEN 2023: Vol 9. Conference Proceedings (pp. 105-114). Association of Economists and Managers of the Balkans. https://doi.org/10.31410/LIMEN.2023.105
References
Aaker, D. A. (1996). Building strong brands. New York: The Free Press, ISBN-0-02-900151-X, pp. 7–25.
Balakrishna, V. K. (1997). Theory And Problems of Graph Theory. The McGraw-Hill. Companies, Inc., ISBN 0-07-005489-4.
Beysolow, T. II. (2018). Applied Natural Language Processing with Python. Publisher Apress, ISBN-13 978-1-4842-3733-5.
Blei, D. M., Ng, A. Y., & Jordan, M. I. (2003). “Latent Dirichlet Allocation”. Journal of Machine Learning Research. 3 (4–5): pp. 993–1022. doi:10.1162/jmlr.2003.3.4-5.993, 12.6.2020
Colladon, F. A. (2018). The Semantic Brand Score. Journal of Business Research, 88 (September 2017), 150 160. https://doi.org/10.1016/j.jbusres.2018.03.026
Colladon, F. A. (2019). Calculating the Semantic Brand Score with Python. Published in Towards Data Science, https://towardsdatascience.com/calculating-the-semanticbrand-score-with-python-3f94fb8372a6
Keller, L. K. (2013). Strategic brand management (4th ed.), Pearson Education Limited, ISBN: 13: 978-0-273-77941-4.
Nitin, H. (2015). NLTK Essentials. Packt Publishing Ltd., ISBN 978-1-78439-690-9.
Sarkar, D. (2016). Text Analytics with Python: A Practical Real-World Approach to Gaining Actionable Insights from Your Data, Apress, ISBN-13 978-1-4842-2388-8.