Albana Gorishti – Faculty of Economy, University of Tirana; Rr. Arben Broci 1 1001, Tirane, Albania
Klaidi Gorishti – Amazon Web Services; Oskar-von-Miller-Ring, 80333 Munich, Germany
Keywords:
Machine learning;
Industrial equipment;
Fog computing;
Classification & Anomaly
Detection
Abstract
The Internet of Things (IoT) concept describes the intelligent connectivity of smart devices using Internet connectivity. In a continuously developing IoT environment, companies try different approaches for predictive maintenance as a solution to reduce costs and the frequency of maintenance activities. Such an environment can natively foster predictive maintenance as it integrates information from different equipment to derive insights and predictions.
This paper proposes a deployment model for predictive maintenance approaches on industrial equipment by processing and analyzing their audio signals. Proper maintenance scheduling is necessary to prevent business costs and maintain the equipment in operational capability.
The authors propose a system architecture to make predictive maintenance applicable in different industrial scenarios. The implementation exploits deep learning neural networks to detect anomalies and further classify them into categories. These machine learning techniques enable predictions of equipment’s conditions and thus maintenance services can be performed
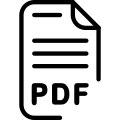
Download file
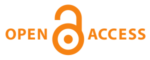
LIMEN Conference
8th International Scientific-Business Conference – LIMEN 2022 – Leadership, Innovation, Management and Economics: Integrated Politics of Research – CONFERENCE PROCEEDINGS, Hybrid (EXE Budapest Center, Budapest, Hungary), December 1, 2022,
LIMEN Conference proceedings published by the Association of Economists and Managers of the Balkans, Belgrade, Serbia
LIMEN Conference 2022 Conference proceedings: ISBN 978-86-80194-66-0, ISSN 2683-6149, DOI: https://doi.org/10.31410/LIMEN.2022
Creative Commons Non Commercial CC BY-NC: This article is distributed under the terms of the Creative Commons Attribution-Non-Commercial 4.0 License (https://creativecommons.org/licenses/by-nc/4.0/) which permits non-commercial use, reproduction and distribution of the work without further permission.
Suggested citation
Gorishti, A., & Gorishti, K. (2022). A Predictive Maintenance Deployment Model for IoT Scenarios. In V. Bevanda (Ed.), International Scientific-Business Conference – LIMEN 2022: Vol 8. Conference proceedings (pp. 129-135). Association of Economists and Managers of the Balkans. https://doi.org/10.31410/LIMEN.2022.129
References
Brügge, B., & Dutoit, A. (2009). Object-oriented software engineering – using UML, patterns and Java (3rd ed.). Prentice Hall.
Dastjerdi, A. V., & Buyya, R. (2016). Fog computing: Helping the Internet of Things realize its potential. IEEE.
Girdhar, P., & Scheffer, C. (2004). Machinery Vibration Analysis & Predictive Maintenance. Newnes, Elsevier.
https://ieeexplore.ieee.org/document/7543455
https://journals.sagepub.com/doi/full/10.1177/1077546316689644
https://www.mdpi.com/1424-8220/18/5/1308
Lee, D., & Pan, R. (2017). Predictive maintenance of complex system with multi-level reliability structure. International Journal of Production Research, pages 4785–4801.
Liebetrau, J., & Grollmisch, S. (2017). Predictive maintenance with airborne sound analysis. Processing Magazine.
Mobley, R. K. (2002). An Introduction to Predictive Maintenance (2nd ed.). Butterworth Heinemann.
Saimurugan, M., & Ramprasad, R. (2017). A dual sensor signal fusion approach for detection of faults in rotating machines. Journal of Vibration and Control.
Simonyan, K., & Zisserman, A. (2014). Very deep convolutional networks for largescale image recognition. ARXIV, cs, arXiv:1409.1556v6.
Yul Oh, D., & Yun, I. (2018). Residual error based anomaly detection using auto-encoder in SMD machine sound. Sensors, 18.
Zhu, X., Kaznady, M., & Hendry, G. (2018). Hearing AI: Getting started with deep learning for audio on Azure. Microsoft.